The ASCAPE projects aims at offering personalized prediction services for quality of life for cancer patients and personalized intervention suggestion services to help to improve their quality of life. The methodology of choice is to train predictive models from patient data using machine learning techniques. ASCAPE aims at closing the full circle starting from a close integration of data collection mechanisms with clinical processes via the development of predictive models and their inclusion and trustful adoption in the clinical practice.
The first challenge of ASCAPE is to have a sufficient amount of data, containing all variables in a coherent format. This is especially challenging because of its target on quality of life and health-related variables, such as physical activity, nutrition or interventions which shall be taken into account going beyond the data that are typically collected in a clinical setting. As a result, the retrospective data which are available at the start of the project is incomplete in that respect, while the prospective data collection needs to start as early as possible to obtain a sufficiently large corpus of data to develop the predictive models
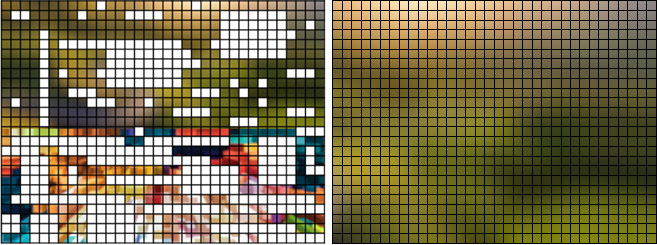
The second challenge is raised from the fact that a portion of the variables are only collected during medical appointments, in unregular time intervals, or their collection depends on the type of treatment a patient undergoes. As a consequence, the datasets are very sparse and many variable fields are unknown and difficult to infer, as they are often categorial and not numeric. Hence, inference or injection of missing values, originating from the analysing of the rest of the entries, needs to be considered much more carefully than in a setting dealing with dense and mostly numerical values as e.g. in image analysis.
The third challenge is that ASCAPE aims at exploring predictive possibilities for quality of life by analysing data that contain more variables than typically collected in a clinical setting. ASCAPE has the asset of having large datasets available at the pilot sites, but this data is incomplete with regard to the whole set of additional variables which the predictive models shall take into account. Hence, the complete data need to be collected prospectively during the project runtime and will be available only in the mature stages of the project. Thus, the the aforementioned data that can be used during the training phase of the predictive models may arrive late in the project.
The fourth challenge results from the handling of personal health data. This challenge raises serious threats to privacy of the patients as no personal or sensitive information on the specific patients shall be disclosed neither directly nor indirectly. Therefore, ASCAPE applies a privacy by design approach by setting up and applying a series of privacy enhancing technologies, which impose challenges to resolve with respect to the project goals. At first, de-identification techniques are applied, which need to be carefully integrated in order to still permit the effective combination of health-records with physical activity information from different sources or geographic-related information from open datasets. Secondly, differential privacy is applied to further reduce the risk of being able to extract information on specific individuals from predictive models, but comes at the price of reduced prediction accuracy. Thirdly, federated learning methods are applied in order to eliminate the need to move unencrypted data from the place where they are collected. The challenge for ASCAPE is that with the aim for an open architecture where further data providing sites may join or leave over time, federated learning mechanisms need to be designed taking into consideration the allowing as such flexibility. Finally, predictive model training algorithms over homomorphic encrypted data will be further improved, in order to allow the collection of encrypted patient datasets in a central place, in which training and usage of predictive models exclusively performed by operating on securely encrypted data.
A final challenge for ASCAPE is that the personalised AI services will indeed be of clinical value. This requires that the predictions and simulations made using the trained models on a specific patient should be conveyed to the medical personnel in a useful and trusted form. The focus here is that beyond the pure results there is especially in the healthcare domain a need to explain the results in a manner that enables medical professionals to understand why and how a prediction has been reached in order to gain trust in the personalised AI services. These explanations need to take the medical domain knowledge and context into account to obtain explanations that are medically useful and provide the necessary statistics used in the medical domain to ascertain results.
Blog post prepared by DFKI